Solar energy is becoming increasingly popular as the world looks to resolve the climate emergency and move toward a greener future. However, solar farms face several energy-generating challenges, including operating and maintenance costs, soiling, performance degradation over time, security and intrusion detection, optimizing energy output, and grid integration challenges.
To address these issues, they increasingly rely on advanced analytics to optimize their operations, monitor weather conditions, and raise maintenance processes. Here, we’ll explore the main challenges faced by solar panels, as well as options for solving them with the help of advanced analytics.
Threshold business analytics approaches cannot deal with the complexity of solar power plant data and the problems that arise. Predictive maintenance, forecasting, and anomaly detection will be the main tools for solving challenges. Solar farms adopting such smart analytics tools have already seen benefits like 12-15% gains in annual energy yield, 15-20% drops in cleaning and maintenance costs, and 30% reductions in power output volatility. By leveraging these tools, solar farms can significantly increase their overall efficiency, reduce downtime, and lower their overall cost of operation.
The article aims to educate readers on the problems and the solutions to aid faster industry adoption of analytics. Continued efforts in this direction can help solar power advance strongly towards its promise of a brighter, cleaner energy future.
Solar Farm Structure
Solar farms consist of solar panels, wires, inverters, batteries, and a utility meter. Due to the design features, each of these areas must be in constantly changing conditions, which causes many problems that require a quick solution.
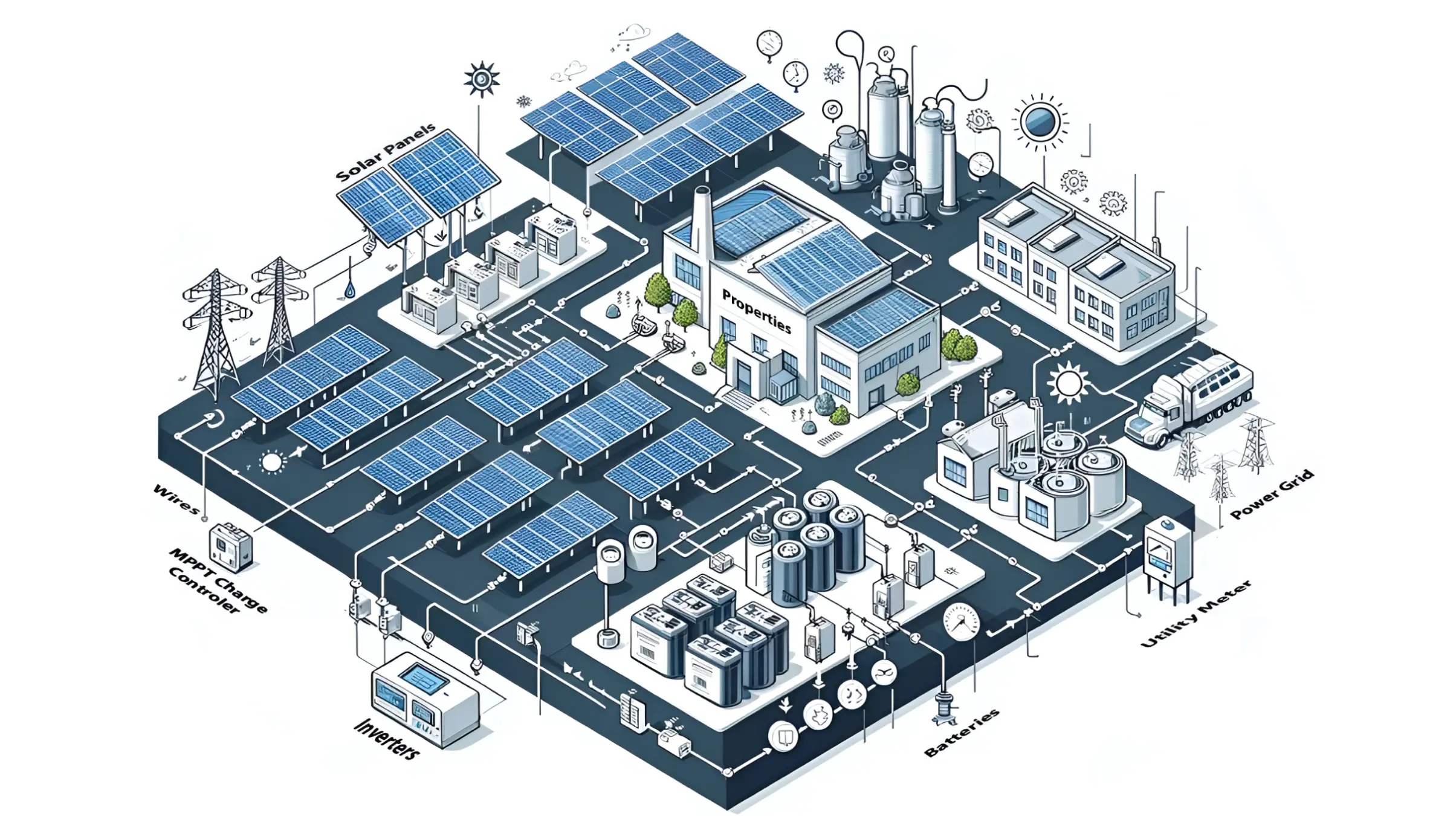
With the help of IoT technologies, we can take indicators that the analytics system can later store, process, and convert into previously unattainable parameters.
Understanding the difficulty of solving challenges
Before identifying the main challenges, it is important to understand their complexity and difficulty. We have identified 3 main causes for the decrease in overall efficiency:
The first is the large number of important metrics that need to be collected for analysis. A solar farm consists of hundreds or even thousands of devices. Each of the devices has a bunch of different parameters that are important for monitoring efficiency. No matter how much we reduce and simplify the system, even a 5 MWh system will have over 10,000 panels. The panels are connected to many inverters and batteries. And they, in turn, are connected by many connections. Accordingly, the system contains a huge amount of data, which is important to operate within a pile.
The second is the instability of parameters due to changing weather conditions and the sensitivity of solar panels to external factors. This makes it impossible to identify patterns and set simple thresholds. A 15% drop in energy production may be due to a passing cloud rather than a breakdown, soiling, or misconfiguration. With parameters constantly shifting, spotting true problems becomes near impossible for standard threshold-based monitoring tools.
The third is the inability of humans to process large amounts of data in real-time to take action to improve the situation. But quick action is essential to maximize productivity. So, the system requires machine intervention when it comes to large arrays of constantly changing indicators.
However, a mature advanced analytics system contains built-in instruments that can help us solve the challenges described. Here are a few of them:
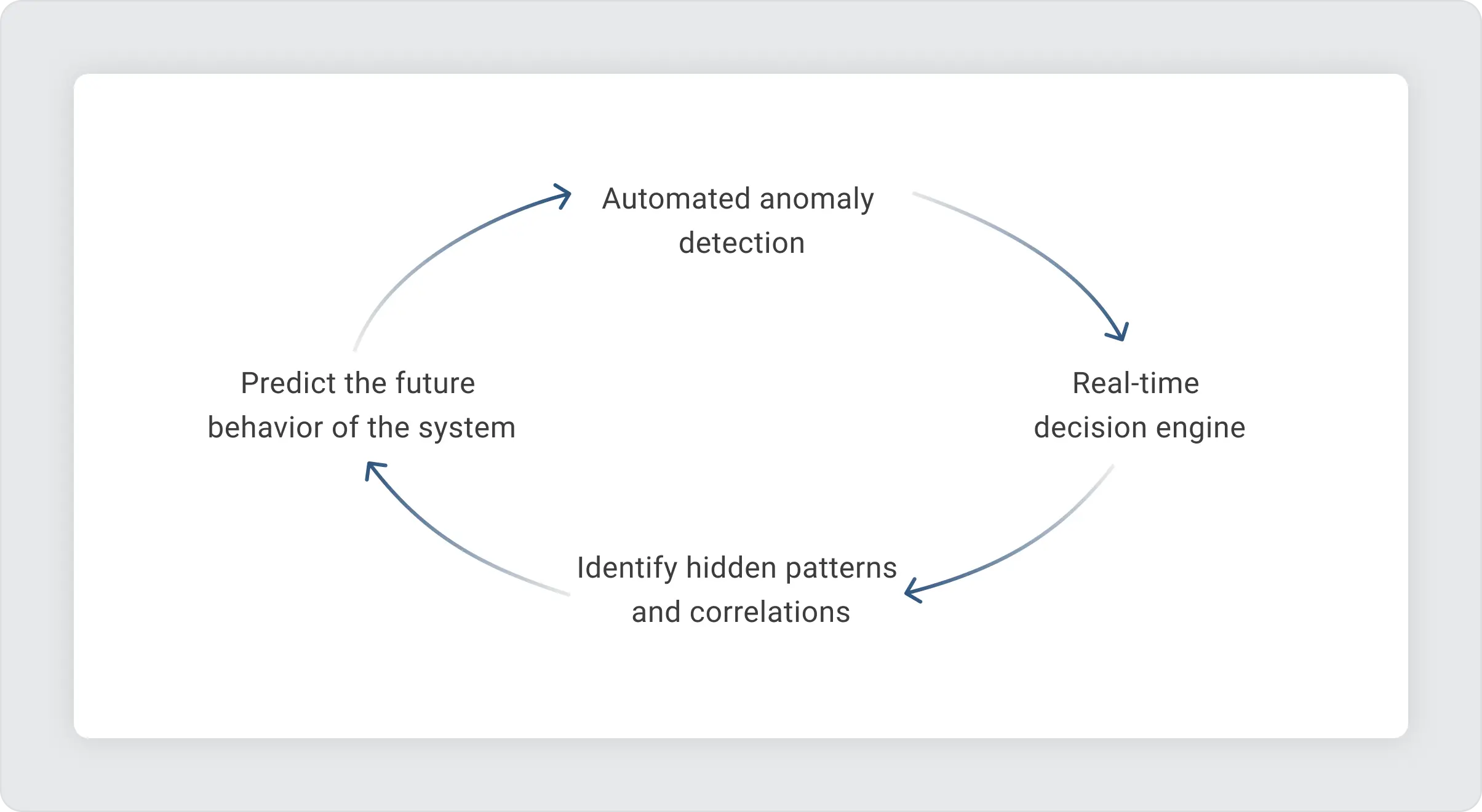
So, advanced analytics can work with large amounts of data, find unusual patterns within it, and make the necessary decisions independently to achieve maximum efficiency.
Now that we understand the complexity of overcoming challenges and the tools that help solve them, we can proceed with a detailed overview of each challenge and possible solutions.
The main challenges in solar farms
Operational and Maintenance Costs
One of the main challenges solar power plant owners face is the significant operation and maintenance costs associated with maintaining these facilities. The main reasons for high expenses are:
- Replacement or maintenance of faulty components
- Panel cleaning
- Equipment wear and tear
- Labor costs
Solar panels are sensitive to many environmental factors, such as temperature, humidity, dust in the air, and other environmental indicators that accumulate over time. Not only do they reduce energy production efficiency, but they also require frequent maintenance and cleaning, which increases overall operating costs.
Here are the most common issues with solar plants that accumulate over time: Delamination and Internal Corrosion, Electrical Issues, Hot Spots, PID Effect, Snail Trail Contamination, Overheating, Isolation Short-Circuit Fault, Inverter Does Not Restart, and MPPT.
If you want to learn more about this topic, see a full description of the problems and their solutions using anomaly detection, and check out the detailed step-by-step guide here.
Expenses such as these can account for up to 15-20% of the total annual cost of solar generation. Traditional maintenance practices often follow a fixed schedule, leading to inefficiencies, unnecessary downtime, and excessive operating costs.
Solar plant managers can use predictive analytics to foresee potential problems before they become costly downtime. This proactive approach minimizes the need for reactive or scheduled maintenance, optimizing the efficiency of the solar plant.
Soiling
The accumulation of dust, dirt, grime, snow, sand deposits, and bird droppings on solar panels blocks incident sunlight to the cells and is a prime source of efficiency losses for solar farms.
Manual cleaning of soiled panels to restore generation is laborious and costly and only offers temporary respite against recurring soiling triggered by environmental or seasonal weather. The high frequency of cleaning needed for farms in specific locales also exponentially increases associated water usage and labor costs. Furthermore, traditional visual inspections for contamination suffer from inaccuracy due to limited manpower and subjective assessments that tend to underestimate early contamination.
Research shows soiling alone can lead to a staggering 7-30% decline in power generation for farms in deserts, near farms, highways, or colder regions. For instance, in some parts of the United States, the soiling rate can range from 0.03 to 0.15% per day, while in desert environments, the soiling loss can be as high as 0.5% per day. These generation gaps for solar farms with tens of thousands of panels placed in arrays covering different geographical regions lead to multimillion-dollar losses.
The way to solve soiling on solar farms with advanced analytics is to use forecasting and comprehensive calculation of the costs of soiling. By finding the perfect balance between the cost of cleaning and the prediction of pollution losses, the system based on real-time data can create a cost-effective cleaning schedule for specific areas.
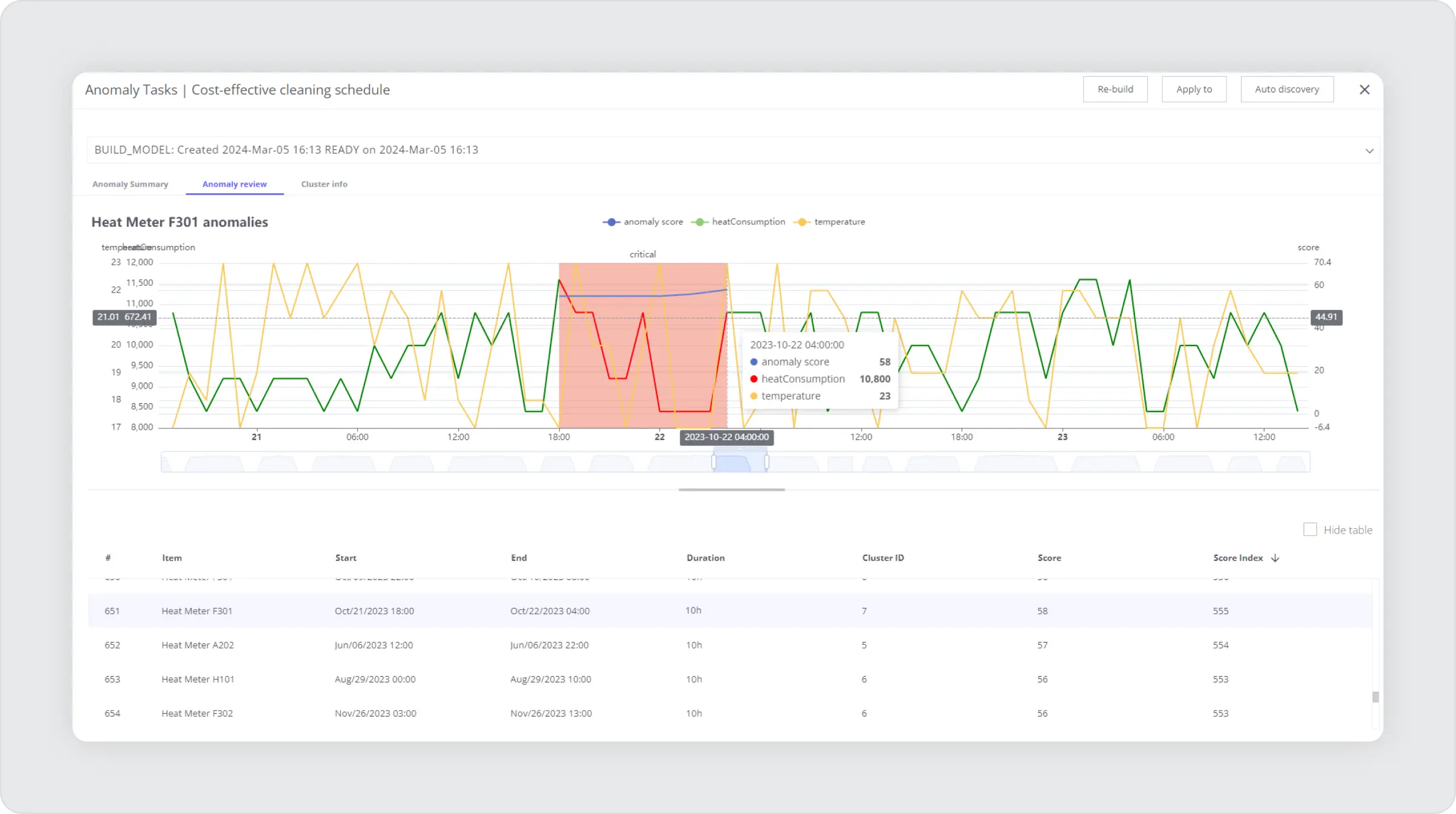
The system can provide a comprehensive report based on actual data, offering an overview of the solar farm’s condition and details about the optimal time for cleaning the solar panels. Additionally, the system can control the activation and deactivation of the nozzles or any other automated cleaning system at the most suitable time.
If you want to learn more about the topic, check out the detailed step-by-step guide covering the topic of “Soiling” in Solar Farms. To read it, click here.
Performance degradation over time
Another problem faced by solar power plant owners is the progressive degradation of generating capacity over the lifetime of a plant, which spans decades.
The long-term impact of natural factors, such as solar radiation, moisture, thermal cycles, and mechanical loads, leads to slow wear and tear of components over many years. Delamination, color change, hot spots, and microcracks in the panels lead to an annual drop in conversion efficiency and electrical output of 0.5-1%.
For a 100 MWh solar power plant, over 25 years of operation, this steady degradation leads to a 10-20% net drop in electricity generation, which means millions in lost profits. Owners also face uncertainty in accurately predicting the degradation trajectories of equipment of different ages located at different sites. False estimates lead to revenue losses due to inaccurate power sales contracts.
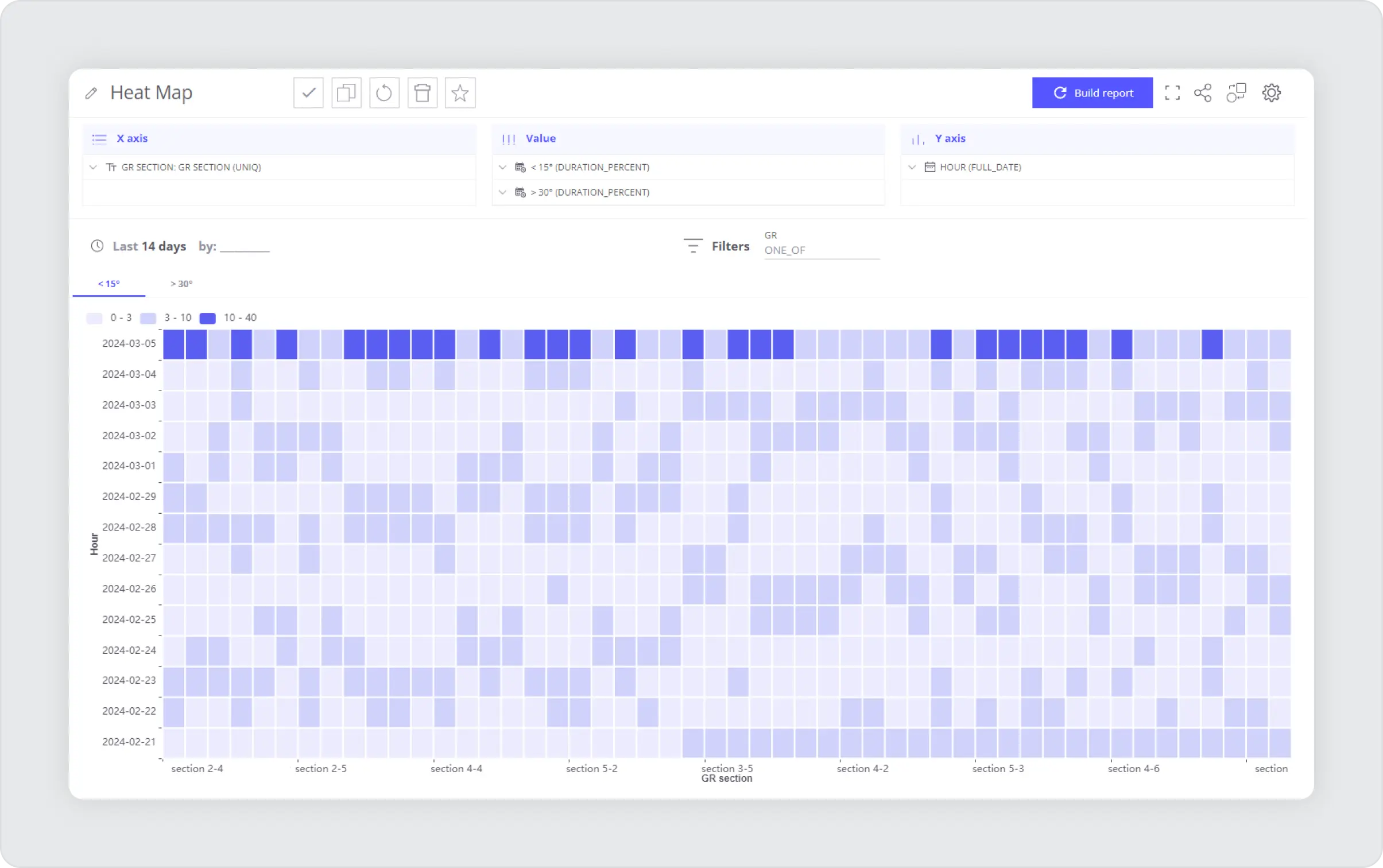
Moreover, solar panels do not only degrade over time. The connection elements, inverters, batteries, and performance monitoring sensors lose effectiveness over time. It should also be noted that because the equipment is open to unplanned complete failure, solar farms may require short- and medium-term replacements.
However, at the same time, we should keep in mind that electricity generation on solar farms is unstable. Therefore, it is not possible to tell the difference between changes in weather conditions and degradation by setting thresholds or simply looking at the indicators.
Advanced analytics allows us to identify long-term and short-term degradation, thus solving this problem. In addition, understanding when the best time to reinvest in equipment replacement is an advantage that will help you maintain the highest possible return on your investment over time.
The system constantly checks indicators from many parts of the solar power plant, making it possible to track the overall condition and degradation of individual areas. Not only is it possible to calculate, but accurate degradation monitoring will help avoid revenue losses caused by unpredictable degradation.
Security and Intrusion Detection
Solar power plants are often located in remote locations and are large installations covering large areas. Therefore, expensive equipment such as inverters and solar panels face significant security and intrusion risks from external intruders. These risks can take many forms, causing huge financial and operational losses.
Theft and vandalism are common, with thieves attempting to steal valuable copper wiring and hackers tampering with energy infrastructure, causing power outages. Natural disasters such as forest fires, storms, and floods also create problems that threaten fencing, cables, and monitoring infrastructure. Additional risks, such as unauthorized access to control rooms or electrical sites through broken gates, also create security risks.
Without reliable monitoring tools, these threats often go undetected for long periods, allowing attackers to cause significant damage. Even traditional legacy security systems, such as motion detectors and surveillance cameras, have blind spots where cunning attackers can bypass detection. Patrolling security also offers inconsistent protection based on a large geography. The lack of real-time context also makes threat response ineffective.
Modern analytics platforms offer comprehensive coverage, analysis, and tools to protect solar power plants better. Sensor data analytics can dynamically monitor multi-factor threats. Algorithms can detect anomalies that indicate potential perimeter breaches. Smart cameras or drones can also detect intruder signals and send alerts to dispatchers faster. The information obtained from the cameras and drones can work in conjunction with other data from the solar farm, giving access to almost complete control over the security of the solar farm. Based on historical data from past interventions, you can build the best strategy to eliminate interference in the future.
Optimizing Energy Output
Solar power plants face the challenge of optimizing energy production due to inconsistent access to their main fuel – sunlight. The energy output changes during the day depending on the sun’s intensity and between days depending on the changing weather conditions.
Complex factors such as cloud cover, temperature, humidity, dust, and vegetation growth also dynamically affect the irradiance falling on the panels.
Starting with potential fines for under-delivery of electricity under power purchase agreements and ending with the impossibility of profitably participating in spot electricity markets based on the fact that there is too much energy to store in batteries of the available capacity. That is why it is important to use complex calculations and forecasts to find the projected energy production for the coming days.
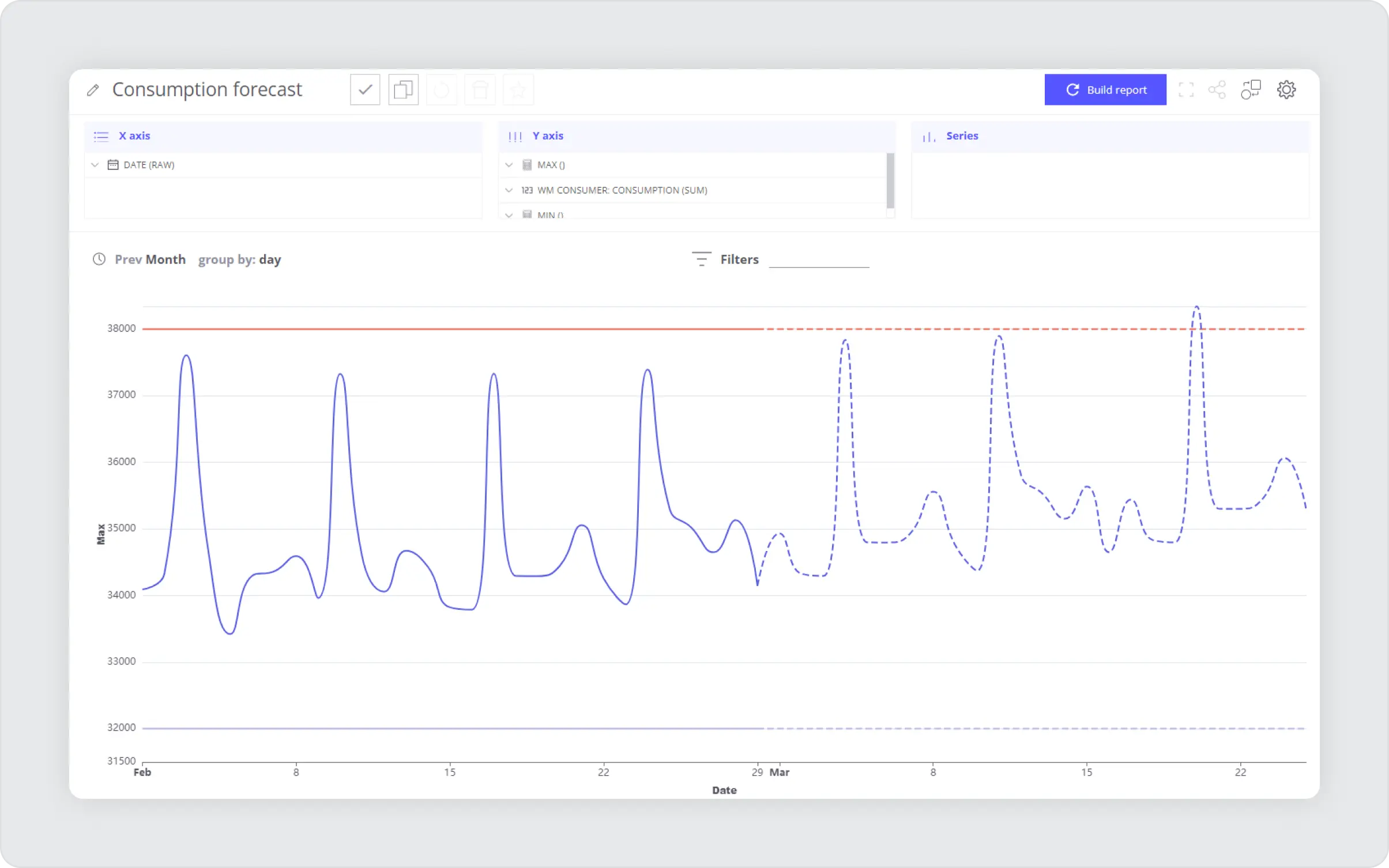
Advanced analytics allows you to detect generation problems by detecting anomalies or forecast problems by predicting them and triggering preventive, corrective actions to eliminate them. The analytical system can also analyze on a minute-by-minute basis whether it is better to take energy from batteries, solar panels, or the system, thus optimizing electricity bills. Such an automated system can significantly reduce electricity bills for households/factories and maximize profits for solar farms.
Grid Integration Challenge
The volatility of solar generation, which is dependent on weather conditions, creates significant challenges for grid integration. Solar energy production changes rapidly depending on cloud cover and time of day, causing voltage and frequency fluctuations for utilities if not appropriately handled. For grids with a high penetration of solar facilities, this variable generation, if increased, can challenge the stability and reliability of the grid.
There are 3 types of system connection to the grid: distributed systems (off-grid), centralized energy (on-grid), and hybrid systems.
- Distributed generation is small solar photovoltaic panels (usually from 5 to 500 kilowatts) installed on residential or commercial properties, and solar panels satisfy the main demand for electricity.
- Centralized generation is large centralized solar parks, usually with a capacity of hundreds of megawatts, which are connected to the power grid via high-voltage power lines. The main difference between off-grid and on-grid systems is that in centralized power generation, all the energy produced goes from the panels to an inverter, which is converted into alternating current and sent almost directly to the utility grid. In a distributed generation, the energy is stored in batteries and used by a residential or commercial facility as needed.
- Hybrid generation differs because it contains batteries and can power a local building and connect to the utility grid.
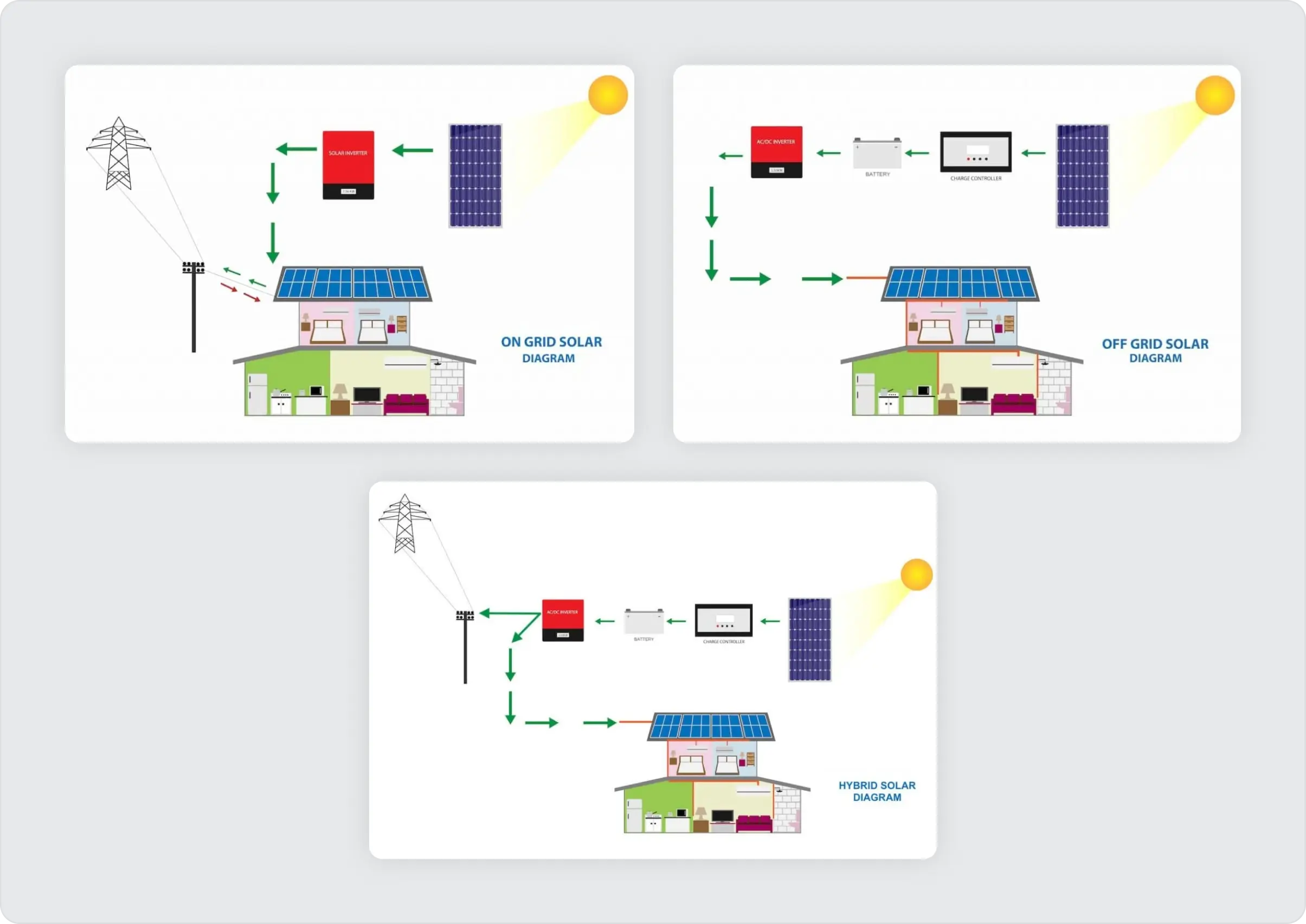
Problems such as voltage spikes, protection discoordination, phase distortion, and unexpected voltage drops can occur when solar power exhibits instability and unpredictability.
Without effective climate change adaptation, problems range from nuisances like flickering lights to catastrophic large-scale blackouts due to cascading power line failures. The economic costs of unreliable renewable generation also reduce investor confidence in further expanding solar power to meet decarbonization goals.
Predictive forecasting combined with optimization for unexpected situations provides actionable information about the future variability of solar generation. Grid operators can take proactive steps to redirect generators, limit the capacity of solar power plants, or deploy batteries to smooth out fluctuations. Real-time anomaly detection also continuously monitors any new equipment limitations or stability risks.
Optimized algorithms prescribe ideal storage vs generation decisions hourly when combined with predictive modeling of dynamic electricity spot prices. Over a year, farms have achieved as much as 5-7% upside in annual revenues besides minimizing deviation penalties.
First steps to solving problems
We realized which problems occur in solar panels. We understood how to solve them with the help of advanced analytics. So finally, let’s define an action plan on how to make it happen with the help of the ThingsBoard IoT platform and Trendz for advanced analytics:
- Collect data from the solar farm site into an IoT platform for processing. Most equipment is capable of reporting data using Modbus, MQTT, HTTP protocols, or gateway data loggers. We need to collect the following information:
- Data from weather stations – solar irradiance, temperature, humidity, etc
- Metrics from Inverters – input and output voltage, current, frequency, etc.
- Metrics from solar panels or Strings – voltage, operating time, panel temperature, panel orientation, etc.
- Metrics from batteries or energy storage systems – voltage and current, state of charge, temperature, etc.
- Information from energy meters and grid – real-time energy flow, power quality data, grid demand response, etc.
- Set up a monitoring dashboard in the IoT platform that will visualize collected data in real-time.
- Configure computation of system-level metrics / KPIs that would describe overall system behavior.
- Train anomaly detection model on historical data to identify abnormal component behavior or equipment misconfiguration. Enable real-time anomaly detection for all on-site equipment.
- Configure predictive maintenance models that would forecast potential issues in the future and compute equipment health scores.
- Set up Alerting and notification rules to trigger automated corrective actions or notify staff about discovered incidents.
Conclusions
Solar farms, due to their design factor, have a large number of challenges that require constant intervention and monitoring of the overall condition of the farm. The main challenges are operating and maintenance costs, soiling, performance degradation over time, security and intrusion detection, optimizing energy output, and grid integration challenges.
Effectively handling these challenges is the basis for the high efficiency of a solar farm.
However, manual reading of indicators or setting thresholds is not enough for power plants with unstable energy production. Using advanced analytics will open up new horizons of efficiency and reliability for your solar farm. The future looks bright for the partnership between solar and advanced analytics!